Designing Autonomous Systems That Are Smarter, Safer, and Solvable
Visitors and sponsors for MARC can expect high-quality research that targets the efficiency, accuracy, and safety of autonomous systems and robots. Our faculty partners with students and external agencies on projects ranging from responsible robotics to real-world systems.
We understand the possibilities and limits of Artificial Intelligence (AI) and the need for testing tech for better usability.
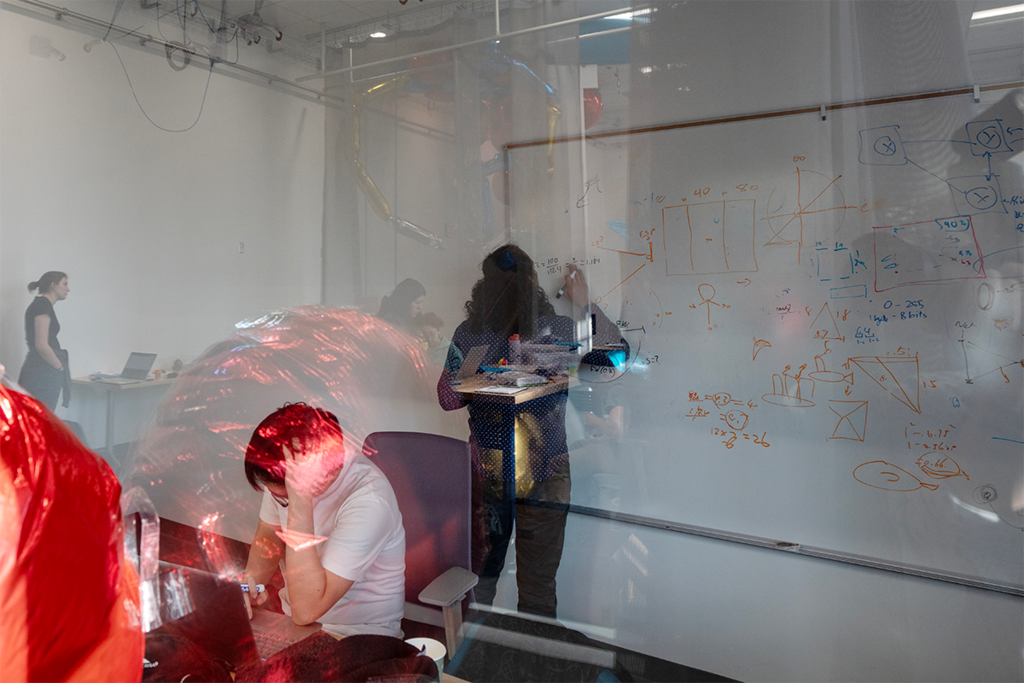
College of Engineering and Computing students in Daigo Shishika's, PhD, Mechanical Engineering Department, Blimp course work together to design and program Blimps in the Research Hall Aviary, Mason Autonomy and Robotics Center (MARC) space on the Fairfax Campus.
Our research encompasses many areas of autonomy and robotics including:
- Human-Robot Interaction
- Sensors
- Distributed Intelligence
- Swarm Robots
- Multi-robot systems
- Computational Statistics
- Data Analytics
- Artificial Intelligence
- Neuromorphic Learning
- Biomedical Robots
- Defense Systems
- Underwater Robotics
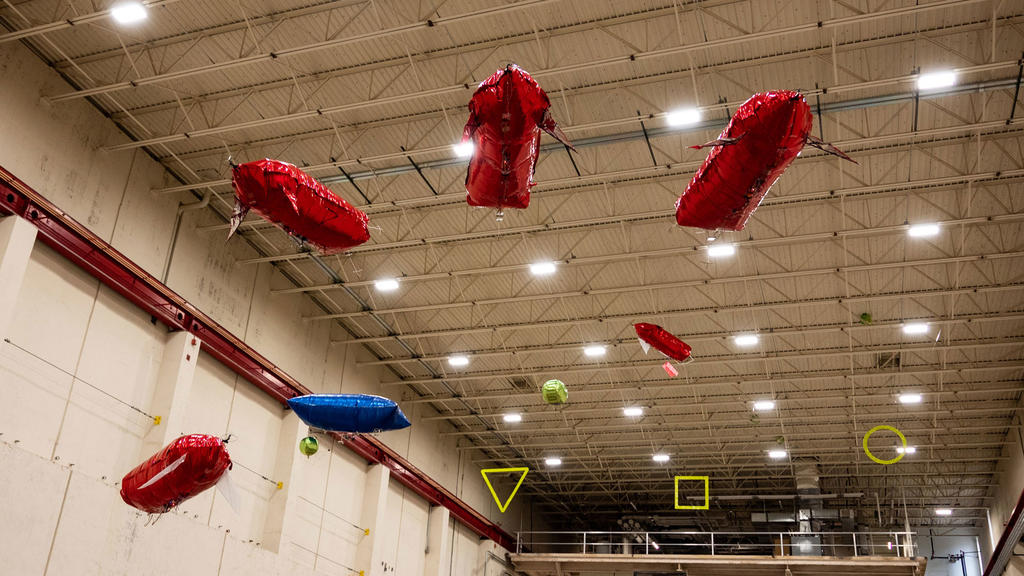
Distributed Intelligence with Lighter-Than-Air Vehicles (2023-present)
This project aims to build robotic agents that can robustly operate in dynamic and contested environments using sensor-actuator pairs that are distributed in the system. The effectiveness of the developed agents is tested and demonstrated by competing in the Defend The Republic competition, which is a Navy-sponsored aerial soccer-game type competition.
Faculty associated with this project: Daigo Shishika and Ningshi Yao.
Studying Human and Robot Co-learning through Human-Blimp Interaction
Presenting the first HRI demonstration between an uninstrumented human and a robotic blimp. Developed a natural interaction between a human and a robotic blimp via human face detection and hand gesture recognition using deep learning.
Faculty associated with this project: Ningshi Yao and Daigo Shishika.
Verti-Wheelers
Improve wheeled mobility in those non-flat environments with vertically challenging terrain, by presenting two-wheeled platforms with little hardware modification compared to conventional wheeled robots. Collect datasets of the wheeled robots crawling over previously non-traversable, vertically challenging terrain to facilitate data-driven mobility. Also, present algorithms and their experimental results show that conventional wheeled robots have the previously unrealized potential of moving through vertically challenging terrain.
Faculty associated with this project: Xuesu Xiao.
Social Robot Navigation
To address this challenge, imitation learning is a promising framework, since it is easier for humans to demonstrate the task of social navigation rather than to formulate reward functions that accurately capture the complex multi-objective setting of social navigation.
Faculty associated with this project: Xuesu Xiao.

Enabling Emergent Behaviors in Unmanned Robotic System Systems (2018-2020)
Rigorously analyze existing distributed algorithms designed for robotic swarm systems and determine whether collisions disrupt the intended behavior of the swarm. The main objective of this project is to determine how robust currently available distributed algorithms are for swarming behaviors when adding the physical property of collisions. A large portion of this project will be dedicated to a search of algorithms designed for physical swarm systems and determining whether enabling collisions inhibits the swarm from achieving its intended emergent behavior.
Faculty associated with this project: Cameron Nowzari.
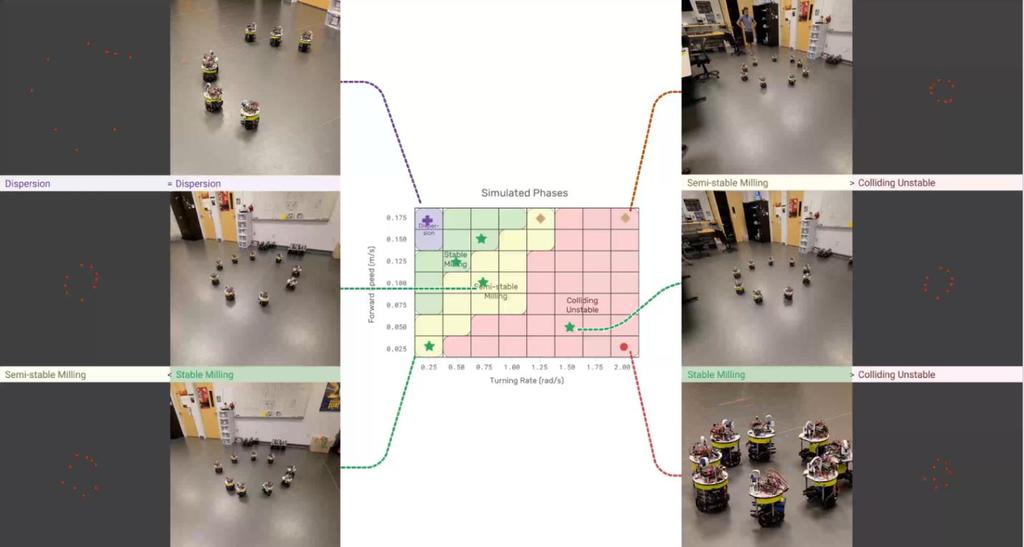
Rapid Design, Development, and Deployment of Robot Swarms via "Good-Enough" Simulations (2022 – Present)
This project takes a bottom-up approach toward the development and deployment of collectively intelligent self-organized robot swarms. Rather than the traditional goal of forcing a particular emergent behavior to occur in an engineered system, we seek to characterize the types of emergent behaviors extremely simple agents naturally exhibit in different environments. More specifically, we will identify real-world verifiable conditions under which certain types of agents in certain types of environments naturally solve different kinds of problems. All theoretical methods and simulations formulated in this project will be in direct support of deploying physical robots in real-world environments.
Faculty associated with this project: Cameron Nowzari, Maryam Parsa, and Sean Luke
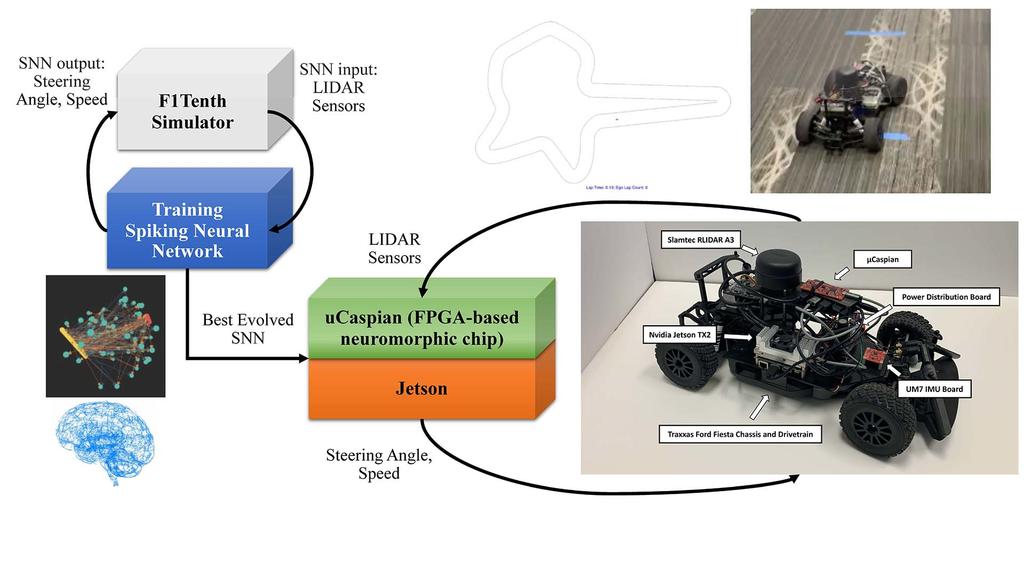
Neuromorphic Learning and Control of Robot Swarms (2023-present)
A transformational breakthrough in the design of robust, adaptable learning algorithms by bridging ideas from neuroscience, brain-inspired machine learning, and swarm robotics. This vision will be realized by establishing a digital simulation-physical experiment connection and showing how this combination of theories can be used to address learning in different dynamic and uncertain environments.
Faculty associated with this project: Cameron Nowzari and Maryam Parsa.